Last month, the FDA approved the first vaccine for chikungunya, a nasty vector-borne infection that can cause fever, chronic joint problems and sometimes death. But unlike the approval of COVID vaccines, this decision was not based on large Phase III randomised controlled trial (RCT) that had tested the efficacy of the vaccine at scale. Instead, the FDA evidence that a certain level of immune response provided a good ‘surrogate of protection’. In other words, some studies had found that the vaccine produced a strong antibody response in humans, while other studies with non-human primates had found that strong antibody responses are generally protective from infection. Together, it suggested that the vaccine would give protection.
Approving a new vaccine based on immunological data is less common than using an RCT looking at efficacy. This is because RCTs require fewer assumptions about immunity: they directly compare infection outcomes in people who get the vaccine and those who don’t. So why was a correlate of protection used for chikungunya? And what does it tell us about epidemic research more generally?
Short on time, short on data
Although chikungunya was first described in 1952, it has become more prominent in recent years. Spread by Aedes mosquitoes, it has caused explosive outbreaks across the tropics. For example, here is the chikungunya epidemic curve (in purple) from an outbreak in the Dominican Republic in 2014:
As you can see, the epidemic only lasts a few months. Which creates a problem for research; by the time someone sets up a large RCT in a hotspot, the outbreak may well be over. And because an RCT of efficacy works by comparing the number of infections in a control group with a vaccinated group, it won’t be able to conclude anything if very few infections occur while the trial is running.
We saw a similar story with Zika. Lots of studies were set up after it spread globally in 2016, but by the time researchers were ready to start collecting data, the epidemics had largely ended. The same challenges occurred during the West Africa Ebola epidemic in 2014-16; lots of trials were set up, but very few succeeded.
Working out the efficacy of chikungunya or Zika vaccines in an RCT isn’t just a matter of running the trial. We’d also need to predict where to run the trial in the first place: an event that will be shaped by combination of travel patterns, climate variability, global levels of infection and immunity (and how accuracy these are being measured), plus a good dose of randomness. Which, unfortunately, puts it beyond the current ability of outbreak forecasting.
The problem is even harder when it comes to infections that occasionally spill over from animal populations. One recent study estimated that the sporadic nature of Nipah outbreaks would mean a traditional RCT would require 43 years and 1.8 million vaccine doses to assess whether the vaccine works.
Beyond vaccines
Vaccines aren’t the only intervention we need to test during emergencies. In spring 2021, I contributed to a scientific framework to inform studies of COVID risk during group events. Despite numerous scientific efforts in 2020, the evidence base for event-specific measures was still quite patchy. Even if multiple measures were used in combination, how much would they reduce transmission in practice?
Answering such questions would not be straightforward. As vaccination levels rose in early 2021, with a vaccine that was highly effective against the circulating Alpha variant, cases were declining. From a research perspective, it wouldn’t be possible to assess the risk of transmission if there weren’t many infections around. (A key reason that COVID vaccines successfully completed efficacy trials so soon in autumn 2020 was resurgent waves in places like US and UK, which meant RCTs accrued information faster.)
As an example, suppose that we want to rule out the possibility that a ‘no intervention’ event without a certain control measure is X times riskier than one with the measure. There are two main things that will influence our ability to conclude this:
1. The value of X we choose. If we pick a large value of X, it will be easier to rule out than a small value. E.g. events that are 10 times riskier will jump out in the data more than events that are only 1.5 times riskier.
2. The level of infection in reality. As we’ve seen for chikungunya and Zika, if only a small percentage of people are infected, the harder it will be to compare the ‘intervention/ and ‘no intervention’ groups. The smaller the percentage, the more people we’d need to recruit into an RCT to spot a difference.
Based on the level of COVID infection in spring 2021 (before the Delta wave), statistical calculations suggested that tens of thousands of participants would have needed to have been recruited into trials to rule out even a two-fold difference in risk (i.e. X=2).
A subsequent paper by some of those involved has some useful reflections on the resulting UK Events Research Programme (ERP) between April and July 2021, pointing out that optimisation of study design and data access is crucial to gather the best quality evidence in a difficult epidemic situation. It also compares the event programme with other aspects of the UK response:
The ERP, despite challenges and compromises, serves as a model for evidence-based policy informing, particularly in emergencies, through a coordinated, adequately resourced, and politically supported science-led research program. This contrasts sharply with the UK COVID-19 Test and Trace program, which lacked impact evaluations and resulted in a lack of high-quality evidence despite a huge budget, reflecting a broader trend of inadequate evaluations in major government projects.
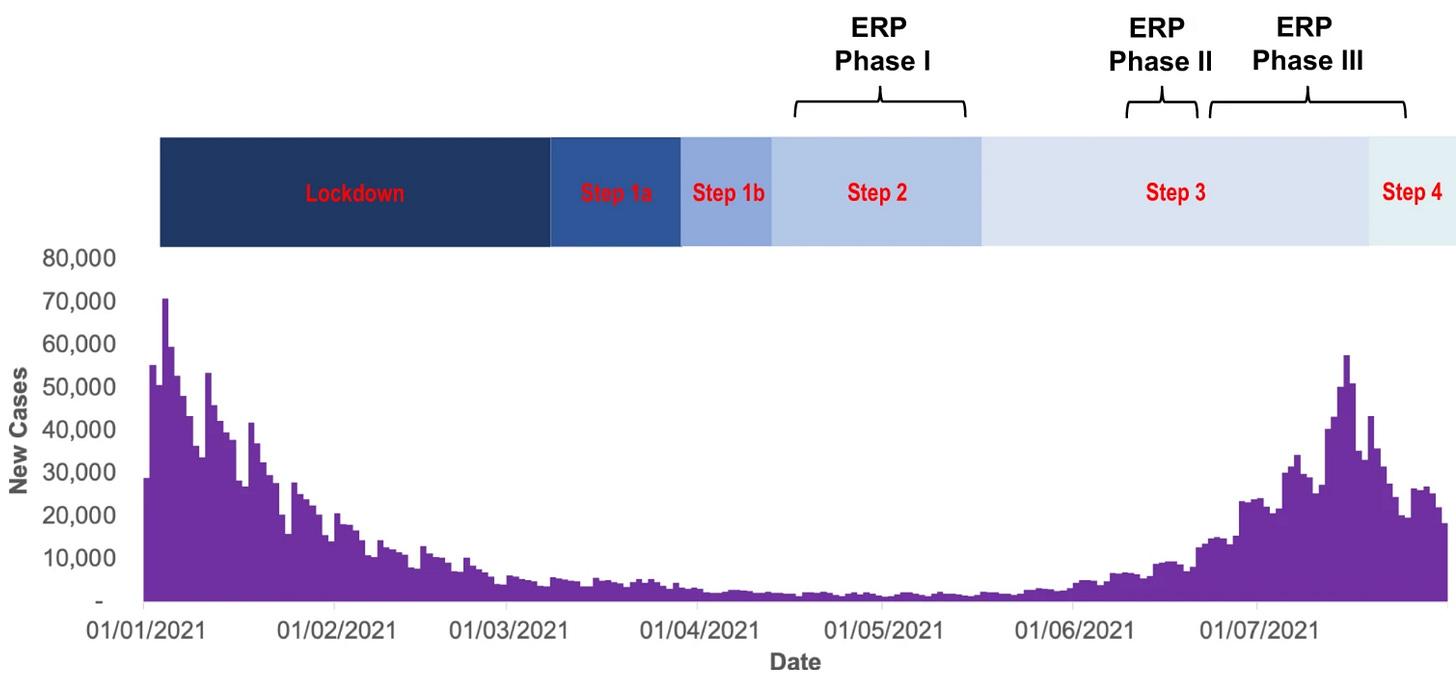
Beyond disease
It’s not just infectious disease research that can struggle to investigate contagion in real-time. Several research projects aimed to study potential misinformation and interference in the 2016 US election, but many of them couldn’t get off the ground in time. Because much of the crucial data has reportedly since been deleted by companies involved, it unlikely we’ll ever have good answers to some of the questions that remain.
Fortunately, has been some progress subsequently, with several detailed studies of 2020 election published earlier this year. Dean Eckles has a nice post with more on the hypotheses that were tested during a project focusing on Instagram and Facebook content.
How can we do better?
The above shows that there are three main types of challenge involved in real-time research:
1. Some events, like the timing of a presidential election, provide a narrow-but-predictable window for research, and some lead time to set up studies. Obstacles to research therefore tend to be mostly about data, privacy and ethics, to ensure the right measurements can be collected and analysed in the event window.
2. Other situations, like a slowly declining epidemic, give a narrow-and-somewhat-predictable window, albeit with not much lead time. This means traditional RCTs will generally struggle to reach a conclusion, and dynamic study designs that account for the transmission process may be more appropriate.
3. Finally, some forms of contagion, like sporadic outbreaks of Chikungunya provide little predictability about either the epidemic window or lead time. This means researchers must either pool information across outbreaks, or find ways to extrapolate conclusions from data that can be collected between outbreaks (like studies of immune responses post-vaccination).
For these reasons, it’s generally much harder to get insights about a short outbreak compared to a stable, slow problem. This is why innovation in this area is so important. It’s bad to suffer a damaging outbreak, but it’s even worse to get to the end of it and not properly understand how to reduce the damage next time.
Adam, most of the time reading your post I feel illuminated and mostly on practical points! thank you and congratulation on your 1000!
Nice discussion of the reasons why RCTs are not always way to study vaccines in certain situations, and sometimes are not possible at all. Thanks for that!