'Flattening the curve' fell flat
In hindsight, it was a terrible piece of science communication
Just over four years ago, a new phrase became common in media coverage: ‘flatten the curve’. It wasn’t a phrase that had appeared much in the epidemiological literature prior to 2020. In part, it seemed to emerge because of the valuable work of data journalists like John Burn-Murdoch, who were documenting the dynamics of cumulative COVID deaths in different countries. Countries that had brought down transmission had curbed the rising tail of deaths, flattening the cumulative curve:
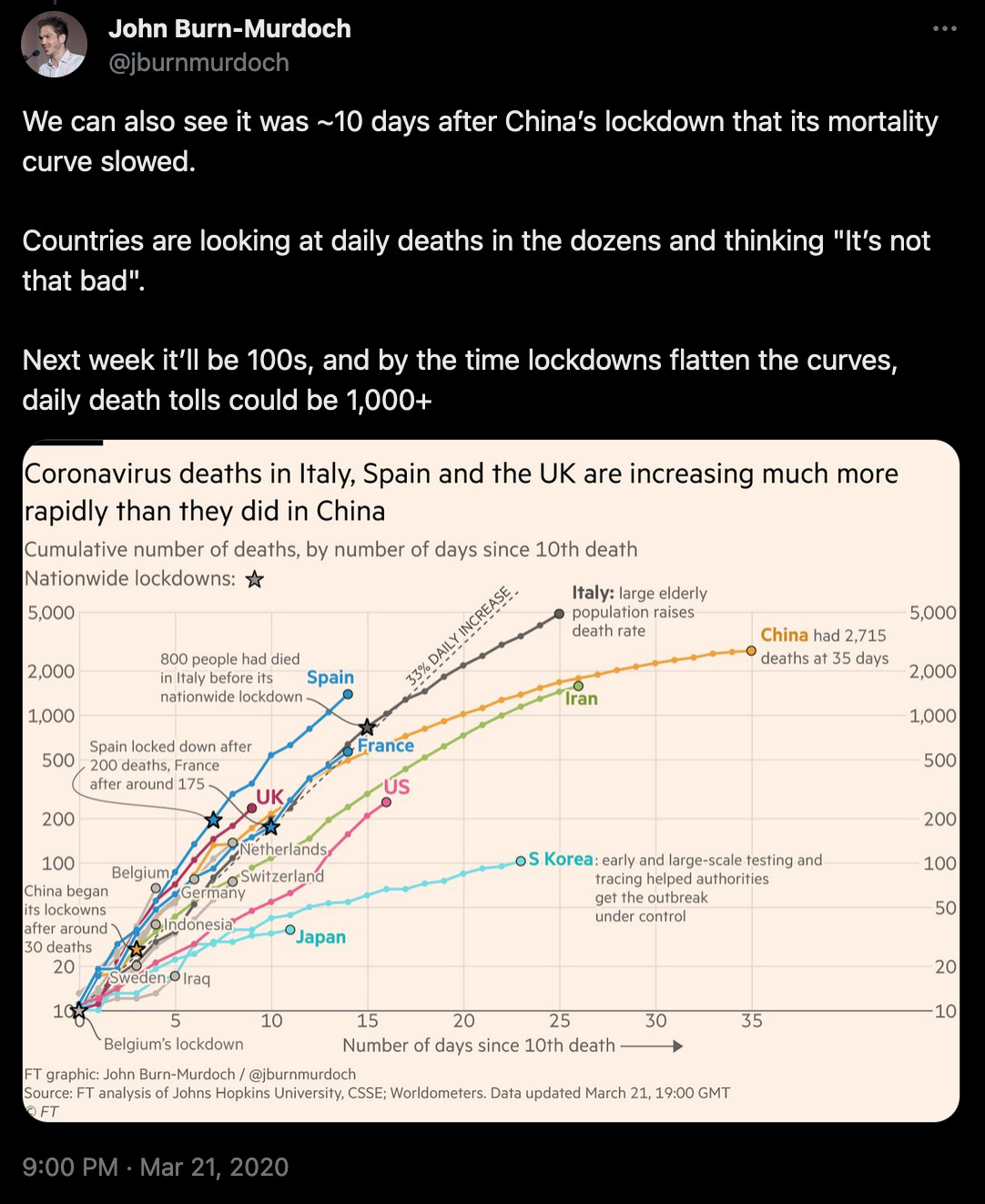
But it was another, slightly different, interpretation of ‘flatten the curve’ that would spread widely during those weeks. Here it had a specific policy implication: if nothing changed, the COVID epidemic would have a large peak of severe cases that would exceed healthcare capacity; but with control measures, the peak would be lower, and remain within capacity.
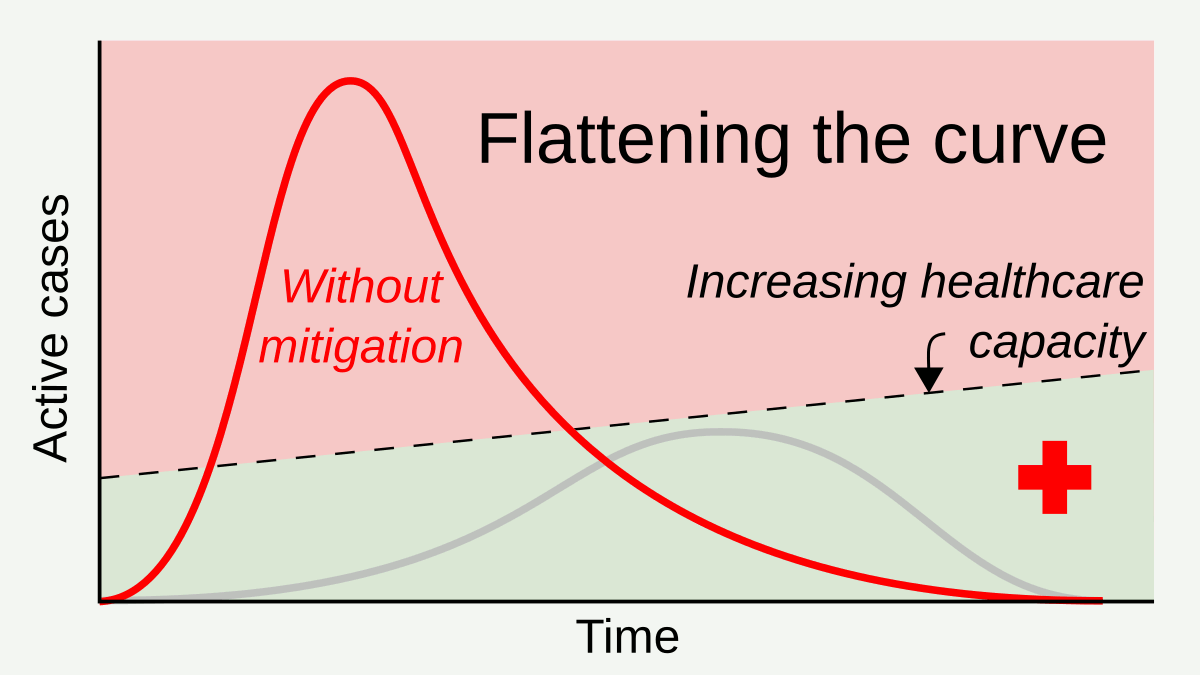
This image was shown widely in March 2020, from government press conferences in the UK to New Zealand. People were looking for ways to helpfully communicate a difficult situation in a relatively simple way, and this graphic became a popular visual aid to answer the ‘why do we need to do something about COVID?’ question.
But there were some big problems with ‘flatten the curve’ as a communication concept. Some of them I had reservations about in early 2020 – and some would become more apparent over time.
A single peak and herd immunity
If someone shows an epidemic with single peak that will be delayed and reduced as a result of control measures, they are implicitly showing an epidemic that ends because of herd immunity (i.e. accumulation of sufficient immunity to tip the epidemic into decline under normal behaviour patterns1).
If an outbreak is pushed into decline solely because of control measures (as happened with SARS-1), then these measures would actually shift the peak earlier. This because control measures have prevented the vast majority of future infections and turned a large, long epidemic into a short, curbed outbreak.
In contrast, if we reduce transmission enough to slow the epidemic – but not enough to tip the epidemic into decline on its own – the epidemic will continue to grow but at this slower rate until accumulation of immunity leads to a smaller, later peak. (For a more detailed explanation, see Principle 2 in this previous post of mine)2.
So if someone shows a ‘flatten the curve’ graph, they are – perhaps without realising – telling the public a herd immunity story.
Herd immunity pre-COVID
There are situations where a population has reached herd immunity during a new epidemic, and predicting this point ahead of time – and the resulting size of the epidemic peak – has been a crucial question.
In 2016, Zika hit the Caribbean island of Martinique. As well as microcephaly, Zika had previously led to cases of Guillain-Barré syndrome, which caused acute paralysis; in severe cases, patients would need mechanical ventilation. The problem was that Martinique only had 8 ventilators.
If the epidemic took the same trajectory as an earlier 2013/14 outbreak in French Polynesia then, adjusting for population size, researchers predicted it was more likely than not that Martinique would need 9 or more ventilators for GBS patients at the peak of the epidemic.
Fortunately, the early growth rate in Martinique was slower than that earlier outbreak. As a result, the updated forecast suggested a maximum of 7 ventilators would be needed, with 3 the most likely requirement at the peak. (In reality, there would be 5 GBS patients on ventilators at the peak.)
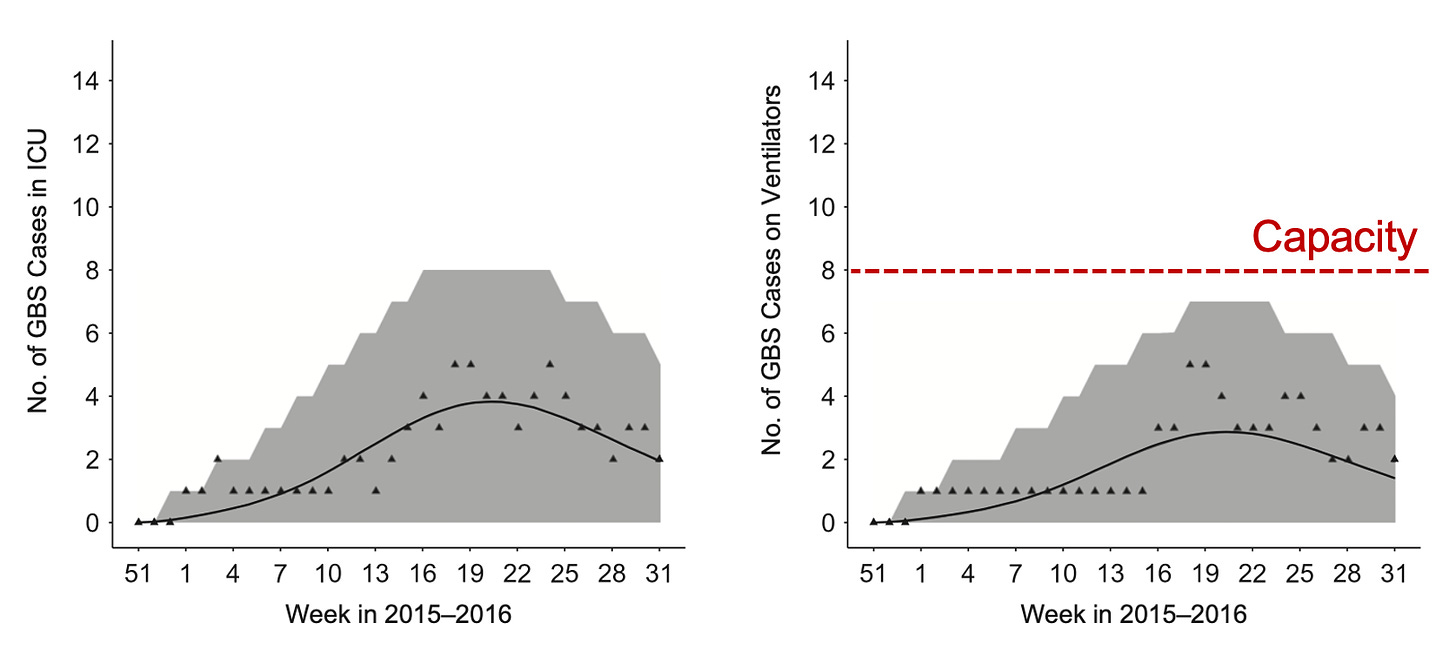
In effect, the local ecology of mosquitoes in Martinique ‘flattened the curve’ relative to what we’d have expected based on the epidemic dynamics in French Polynesia. It was a textbook case of a smaller, later peak that still ended because of accumulation of immunity.
Not nearly flat enough
The problem with COVID was that the epidemic of severe disease was going to be far too big. In early 2020, the UK ICU bed capacity was just under 5000 beds. Early modelling analysis by our group and others suggested that in the scenario where an epidemic was sufficiently large to generate enough immunity to tip transmission into decline, the NHS capacity would be exceeded many times over3.
Note that subsequent data point to the same conclusion. During the spring 2020 peak, around 3200 patients were in ICU with COVID. And around 7% had evidence of past infection by end of May 2020 (and much less than this would have been infected at the peak). So if the epidemic were to peak once half of the population got infected, for example, we’d have plausibly been looking at tens of thousands of patients in ICU at this point.
Flattening the curve – with a smaller, later peak within healthcare limits – was an imaginary reality, which was never going to happen. But the effects of the picture were very real. I think it inadvertently anchored many people to the idea of a single peak, and with it the idea it would all be over after that first wave. I remember the scepticism I faced (and many others also faced) when trying to point out the potential for an awful second wave. It wasn’t helped by widespread coverage of the ‘flatten the curve’ image, with no follow-up wave in sight.
Most countries introduced lockdowns with no exit strategy. Rather than focusing on the key problem (i.e. ‘We are in a lot of trouble with horrible trade offs and we need to find a way out of it’) I wonder if that single-peak image lulled some into complacency (‘How bad will it be really? Haven’t we had the big peak already?’)
The area under the curve matters as well as peak
The second wave that started in the UK in late 2020 and swept through in early 2021 was horrific. To give just one example, here is the experience recalled by a consultant surgeon:
I worked as an Intensive Care Unit nurse in the Jan 2021 surge for 5 weeks. That was more traumatic than having COVID. My ICU colleagues were burnt out, clinically depressed, and crying at work. I do not know how they did this for two years.
During autumn 2020, graphs often showed the height of the spring 2020 peak for reference. But these were are arbitrary numbers to get anchored to, and ones that would have seemed horrifying in late 2019.
Anyway, it wasn’t just the height of the peak that mattered; it was also the area under the curve. The winter Alpha variant wave had a similar peak number of deaths as the first wave, but it was broader because it came on the back of an autumn resurgence with hundreds of daily deaths.
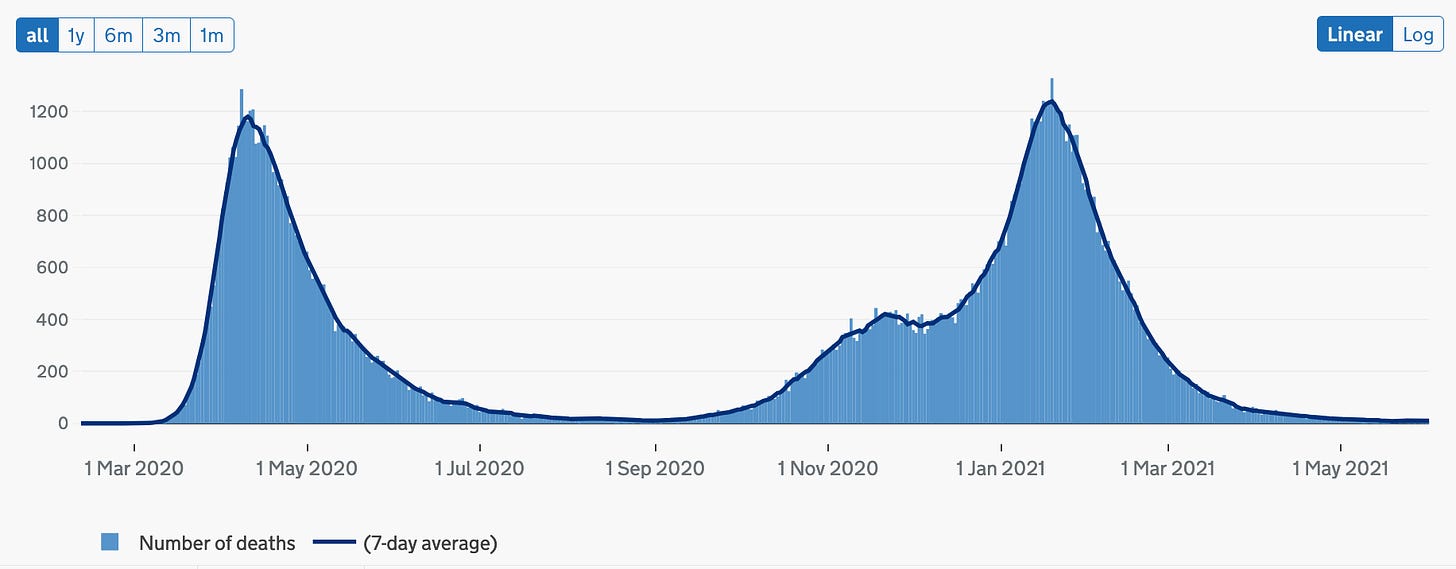
During the subsequent Delta wave, we saw a similar focus on the size of the potential peak ahead of the July reopening. But rather than a short, sharp epidemic, we instead saw residual caution in the population post-reopening, and hence a long plateau at around a hundred deaths a day:
In effect, the slow return to normal behaviour had ‘flattened’ the Delta wave. But because coverage had become so anchored to the size of that spring 2020 peak – the one people had been so encouraged to focus on in those ‘flattening the curve’ pictures – there was much less discussion of the cumulative numbers that autumn. And on the remaining gaps in vaccine coverage that were contributing to them.
Focus on susceptibility, not just single peaks
Although I wasn’t that happy with the term ‘flattening the curve’ in early 2020, I did use it occasionally at the time. Those graphs of cumulative deaths levelling off in South Korea and China were being widely shared, and it seemed helpful to use familiar language to describe the situation.
But was the ease of a short-term soundbite worth the longer term damage to understanding? Were we at risk of telling too much of a public health ‘white lie’ – communicating the idea that transmission needed to be reduced, but at the price of miscommunicating the situation countries were actually facing?
How to convey COVID dynamics in an easy-to-grasp but accurate way was something I grappled with a lot in 2020. One realisation I had was to focus on susceptibility rather than just the shape of the epidemic. By late February, we had a good idea of what the infection fatality risk was in different age groups (despite the best efforts of some commentators to pretend otherwise). So we could estimate how many people we’d expect to have a given outcome (e.g. symptoms, ICU admission, death) if they were infected.
In interviews, framing the problem around susceptibility make it easier to communicate where we were and where we were going. Lockdowns and other reductions in contacts could temporarily reduce transmission but wouldn’t change susceptibility. The first wave might end, but if we went back to normal, the conditions were still ripe for another one. Vaccines or treatments – or a very large, damaging second wave – was the only way this pandemic was going to end. As I put it in one interview in mid-March 2020: ‘Whatever we do, we’re potentially going to have to face for a year or two.’
I made that statement in a few interviews during that period, and each time the interviewer was taken aback. A year or two? The ‘flattening the curve’ graphic didn’t show that. But I’d come to appreciate that oversimplifications weren’t helpful, and were increasingly damaging. We had to find a way to be usefully frank with the public. Despite all the uncertainty, people needed a conceptual toolkit to process the situation we were facing – and the situation we would continue to face into the future.
If you’re interested, I wrote more about communication in public health here:
Some commentators have tried to redefine the term ‘herd immunity’ during COVID (e.g. to mean ‘epidemic disappears forever’), but the definition in the above text is the established epidemiological one, which was familiar to researchers working on epidemic dynamics pre-COVID.
It’s worth noting that the area under the curve will be smaller if transmission is lower, because it reduces the final size of the epidemic, and the final size is larger than the herd immunity threshold. In effect, flattening the curve reduces the ‘overshoot’ effect I described in this post. For example, it’s been estimated that the reduction in social contacts during school holidays reduced the size of the 2009 influenza pandemic in the UK by around 20%.
We also considered a scenario where the most at risk age groups reduced their contacts and immunity accumulates in the rest of the population, as some people were belatedly also suggesting in in autumn 2020. But the problem is that there were just too many people at risk of severe outcomes in 2020 (almost 1 in 5 people are over 65 in the UK) and they are too socially embedded with the rest of the population to keep infection away from them during a massive epidemic.
Great article. But I guess the whole 'flatten the curve' cycle was shaped by politics. Most leaders would see the 'year or two' message as political suicide.
Adam, this is excellenet!
Thank you!